Personalised Care Interventions: Rapid Evidence Review (diabetes, MSK & COPD) Report
Contents
Undertaken by Transformation Partners in Health and Care, part of the Royal Free London NHS Foundation Trust
Report prepared by:
- Rhys Ellis
- Dr Alex Mears
With academic support from:
- Hayley McBain (SW AHSN)
- James Hayward (NHSE DAIS team)
And clinical SME support from:
- Karla Sypsa (diabetes)
- Afroza Shamsudin (MSK) Kara Renno (COPD)
Introduction
The Personalised Care (PC) agenda is a key part of the NHS Long Term Plan (NHS, 2019), and will be part of Business as Usual for NHS care going forward. The aim of this policy is to embed personalised, person-centred care into all local health systems. The Universal Model of PC includes six components (NHS 2019(2)):
- Shared decision making (SDM): People are supported to understand the care, treatment, and support options available and the risks, benefits and consequences of those options, and to make a decision about a preferred course of action, based on evidence-based, good quality information and their personal preferences.
- Personalised care and support planning (PCSP): People have proactive, personalised conversations which focus on what matters to them, delivered through a six-stage process and paying attention to their clinical needs as well as their wider health and wellbeing.
- Enabling choice, including legal rights to choice: Enables choice of provider and services that better meet people’s needs, including legal rights to choice in respect of first outpatient appointments, and suitable alternative provider if people are not able to access certain services within the national waiting time standards.
- Social prescribing (SP) and community-based support: Enables all local agencies to refer people to a ‘link worker’ to connect them into community-based support, building on what matters to the person as identified through shared decision making / personalised care and support planning, and making the most of community and informal support.
- Supported self-management (SSM): Increasing the knowledge, skills and confidence (patient activation) a person has in managing their own health and care through systematically putting in place interventions such as health coaching, self-management education and peer support.
- Personal health budgets (PHB) and integrated personal budgets (IPB): An amount of money to support a person’s identified health and wellbeing needs, planned and agreed between them and their local Clinical Commissioning Group. May lead to integrated personal budgets for those with both health and social care needs. This isn’t new money, but a different way of spending health funding to meet the needs of an individual.
As with all important policies, it is crucial to establish and maintain the evidence base for the interventions prescribed. Much research has been undertaken into the effectiveness of PC, but it has been sporadic and has grown piecemeal. While previous evidence reviews have been undertaken, there are gaps, mostly with regard to the evidence around impact on system utilisation, such as hospitalisation, use of A&E and GP appointments.
The key policy group for this work is the NHS Evidence task and Finish Group, part of the Primary care, community health services and personalised care team at NHS England. This group sought to commission a piece of work to address the gaps (Appendix 1). The commissioners of the review have requested the team look specifically at three key condition areas (diabetes, MSK and COPD), with a focus on literature in three areas: strong evidence base, a key role in improving health, and impact to current priorities.
Background
There have been previous reviews of the impact of Personalised Care, which have been used to inform our approach. Three key examples are:
- Personalised Care Group Evidence Review 2019/20. Undertaken by the NHS Data, Analysis and Intelligence Service (DAIS).
This paper reviewed the evidence for each of the components of PC in turn. For shared decision-making (SDM), there was substantial evidence that there is potential for improvements in developing a supportive health care system and enabling NHS workforce. The main impacts are on patient experience, but there is no new evidence of impacts on the system. For SSM, the evidence again was strong for improved health and wellbeing outcomes, with implications for system design. PCSPs were found to aid mental and physical recovery. Community-based approaches showed limited and mixed support.
The best support in the evidence was found for group-based self-management, disease-specific self-management education, self-monitoring, online education, and telehealth. The outcomes for these interventions focussed, again, on patient health and wellbeing rather than system impact.
Mental and physical health and well-being: Person- and community-centred approaches have been shown to increase people’s self-efficacy and confidence to manage their health and care, improve health outcomes and experience, reduce social isolation and loneliness, and build community capacity and resilience.
NHS sustainability: These approaches can impact how people use health and care services – and in particular can lead to reduced demand on services, particularly emergency admissions and A&E visits.
Wider social outcomes: Person- and community-centred approaches can lead to a wide range of social outcomes, from improving employment prospects and school attendance to increasing volunteering. They also can potentially contribute to reducing health inequalities for individuals and communities.
At this point, we can outline the aim and objectives for this project.
Aim
The aim of this project is to provide a report outlining the breadth and depth of evidence to support the deployment of personalised care interventions to reduce healthcare utilisation in all parts of the healthcare system.
Objectives
- To develop a methodology to identify all relevant literature and sift to yield a shortlist of the most high-quality, probative papers
- To review the shortlist of papers to draw out key messages and key numerical findings
- To synthesise these key messages to identify the key themes across the literature
- To draw out the system and policy implications of these key themes
Methodology
Approach
Given constraints on time and resource, there is no possibility to undertake a full systematic review. In addition, the literature in this area is broad and highly complex. For these reasons, we have chosen to focus on previously undertake systematic reviews and meta-analyses, rather than primary research (Smith et al, 2011).
This evidence synthesis takes the form of a Rapid Evidence Assessment (REA) (Watt et al, 2008). An REA is a tool in the systematic review methods family and is based on comprehensive electronic searches of appropriate databases, internet sources and follow-up of cited references. To complete REAs in a short timeframe, researchers make some concessions in comparison with a full systematic review. Exhaustive hand searching of journals and textbooks is not undertaken, and searching of ‘grey’ literature is necessarily curtailed. This shortened timeframe is essential for policymakers to meet deadlines, but increases the risk of publication bias. In this case, the review process has been carefully developed and planned to reduce biases and eliminate irrelevant studies.
In order to undertake such a review, we deployed a validated and tested tools to aid us. The chosen tool aids identification of the key components of the research question and is endorsed by the Cochrane Collaboration (Higgins & Green, 2013).
The tool is called PICO, and this breaks the research question into four elements: Patient or Population, Interventions, Comparison and Outcomes of interest. Using these elements, it is a far more straightforward task to build a search strategy and draft a strong research question.
Using PICO as the structure, we formed the research question below, as refined post engagement with key stakeholders:
‘In those with MSK, diabetes or respiratory disease (P), what has been the effect of “Personalised Care” [as defined by the universal model of personalised care] (I) on health utilisation, clinical outcomes, wellbeing outcomes, patient experience & safety (O)?’
In accordance with an accepted extension, we added S to PICO to make PICOS, where the S refers to the study design. This allowed us to further refine our search strategy (Methley et al, 2014).
Exclusions
In order to i) maintain the focus of the review and ii) reduce the number of studies to a manageable level, we used some specific exclusion criteria:
- Papers more than ten years old. Personalised Care and its components were less likely to have been studied outside this timeframe, and research in many cases has been superseded as theory and evidence move on.
- Papers which aren’t a systematic review. Due to time constraints, we believed this study design provided the most value for our research.
- Findings lacking relevance to the research question. With many papers to review and a limited resource, it is important to focus on those studies directly relevant to the research question.
- Studies lacking probative value. As an effectiveness review (with a clear mandate from the PCG), we excluded papers that did not tend to offer evidence as to the effectiveness of interventions, in whichever direction.
- Papers of low quality. We excluded papers where the methodology or findings were weak or lacked evidential basis.
Data sources and search strategies
Our search strategy included alternative terminology and subject headings (/), combined with Boolean operators (AND, OR, ADJN), truncations (*), wildcards (?) and the Explode or Focus function (exp), allowing us to expand the scope of our search.
Terms were informed by the NHS Long Term Plan, discussions with subject matter experts and the synonym function within the databases. We refined our use of subject headings, truncations, and terminology as part of an iterative search process to obtain a manageable number of studies for review.
A full table of search terms is available at Appendix 1.
Searches were performed in Embase, Medline and The Cochrane Library. These three were chosen to maximise the coverage we would achieve, and would include journals focussing on social care, not just health.
This was supplemented by a review of relevant NICE Guidance.
This search strategy left us with the following literature:
Condition | Medline | Embase | Cochrane | Total | Total (post de-duplication) |
---|---|---|---|---|---|
MSK | 132 | 377 | 3 | 448 | 304 |
Diabetes | 272 | 322 | 5 | 599 | 407 |
COPD | 73 | 89 | 2 | 146 | 129 |
Review selection
A robust screening process removed irrelevant articles and duplicates. This took place in four stages:
Initial screening
We screened and scored literature titles between 1-4 in relation to their relevance to our research question. 1 being of no relevance and 4 being very relevant. Titles with a score of 2 or more were considered for the longlist.
Longlist screening
We developed an excel formula to identify and count the occurrence of any specified words within a study abstract. This program was used to determine which studies had the strongest connection to personalized care and allowed us to identify systematic reviews that met our inclusion criteria.
Shortlist screening
The remaining papers were screened by abstract to ensure they were relevant in answering our research question.
Full-text screening
Whilst completing a full text review, we extracted key data from the papers, assessing the strength of argument and quality of evidence.
Final funnelling outcomes
Stage 1: Search the databases
- Papers found:
- Diabetes = 407
- MSK = 304
- COPD = 129
Stage 2: Initial screening
- Papers left:
- Diabetes = 258
- MSK = 155
- COPD = 95
Stage 3: longlist screening
- Papers left:
- Diabetes = 33
- MSK = 19
- COPD = 13
Stage 4: Shortlisting
- Diabetes = 19
- MSK = 15
- COPD = 6
Quality assessment
We used the AMSTAR 2 (A MeaSurement Tool to Assess systematic Reviews; Shea et al, 2017) tool to rate the quality of the included systematic review (This tool provides a robust, systematic framework for the assessment of systematic reviews. The tool gives four levels of confidence rating:
- High: No or one non-critical weakness: the systematic review provides an accurate and comprehensive summary of the results of the available studies that address the question of interest
- Moderate: More than one non-critical weakness*: the systematic review has more than one weakness but no critical flaws. It may provide an accurate summary of the results of the available studies that were included in the review
- Low: One critical flaw with or without non-critical weaknesses: the review has a critical flaw and may not provide an accurate and comprehensive summary of the available studies that address the question of interest
- Critically low: More than one critical flaw with or without non-critical weaknesses: the review has more than one critical flaw and should not be relied on to provide an accurate and comprehensive summary of the available studies
The AMSTAR 2 assessment tool has sixteen items, however 7 of these are classed as critical to review quality:
- Protocol registered before commencement of the review (item 2)
- Adequacy of the literature search (item 4)
- Justification for excluding individual studies (item 7)
- Risk of bias from individual studies being included in the review (item 9)
- Appropriateness of meta-analytical methods (item 11)
- Consideration of risk of bias when interpreting the results of the review (item 13)
- Assessment of presence and likely impact of publication bias (item 15)
Data extraction
Each paper within the full text review was read and notes were taken to capture:
- Age
- Study Design
- Intervention
- Population
- Outcomes
- Participants (n)
- Studies (n)
- Results
- Mediating Factors
- Setting
- Key Takeaways
- Lessons for Implementation
- Appraisal
- AMSTAR Score
NICE Guidelines
The process for development of Clinical Guidelines (NICE, 2022) is seen as the gold standard for systematic reviewing, and we therefore undertook an additional review of relevant Guidelines.
The following Guidelines were identified as relevant:
- Relevant Guidelines for diabetes:
- T1 in adults
- T2 management in adults
- T2 prevention
- Relevant Guidelines for MSK:
- Osteoarthritis >16
- Chronic pain >16 factors
- Chronic pain >16 communication
- Chronic pain >16 pain management programmes
- Chronic pain >16 social interventions
- Relevant Guidelines for COPD:
- COPD >16
The reference list of evidence included for each guideline was captured, and the abstracts searched for any relevant keywords. Papers were excluded if they were >10 years old, not UK based, not relevant to Personalised Care, or not probative for our research question.
In total, 110 papers included the keywords, and 14 passed the exclusion criteria. Two were already within our screened list, and seven more passed the final screening.
Synthesis
This review took a narrative effectiveness synthesis approach. As part of the synthesise process, key information in the studies were identified, extracted, and coded as either a clinical, wellbeing, patient experience, patient safety, or healthcare utilisation outcomes.
During the data extraction and synthesis process, we also assigned additional tags to key information within the literature, covering areas such as intervention details, the topic/theme discussed, and the levels of effect.
This would go on to inform our synthesise table, an excel based database which provided us with added functionality to analyse the impacts of personalised care. Once all data was extracted, we manipulated the data in the synthesis table to identify key themes, findings, and mediating factors within the body of research.
Results
Assessment of review quality
Quality Assessment: AMSTAR 2
The AMSTAR 2 (A Measurement Tool to Assess Systematic Reviews) checklist is a tool designed to assess the methodological quality of systematic reviews. It consists of 16 items that evaluate various aspects of a systematic review, including the research questions and inclusion criteria, establishment of review methods, study selection and data extraction procedures, risk of bias assessment, data synthesis, and publication bias investigation, among others. The checklist is intended to help researchers and reviewers evaluate the quality of a systematic review and identify any potential methodological weaknesses or biases that may affect the validity and reliability of its findings.
As all our included studies are either Systematic Reviews or Meta-Analysis, this influenced our decision to use the AMSTAR2 Checklist as our quality assessment tool. To provide a comparison, we allocated a weighting scores to each of the AMSTAR 2 outcomes. Weighted scores were added and divided by the number of papers to give a mean.
Condition area | Critically low (weighting 1) | Low (Weighting 2) | Moderate (Weighting 3) | High (Weighting 4) | Adjusted score (mean) |
---|---|---|---|---|---|
Diabetes | 0 | 4 | 7 | 8 | 3.21 |
MSK | 2 | 0 | 3 | 4 | 3 |
COPD | 0 | 0 | 0 | 5 | 4 |
If we were to compare the quality of studies, COPD has the highest scoring literature when assessing against the AMSTAR2 checklist. However, COPD also had the fewest number of studies included. The largest evidence base was around diabetes and personalised care interventions, providing us enough data to group the studies by broad intervention types.
Diabetes:
Variability in the papers
- Eleven systematic reviews and eight meta-analysis
- participant numbers ranged from 53 to 4097
- number of included studies ranged from 3 to 232
- variety of settings and patient demographics
- papers mostly looked at SSM, some SDM and one Social Prescribing
- variety of interventions used
Areas of commonality
- All patients with diabetes (type 1 and type 2)
- Often used Hba1C as the primary clinical outcome measure
- Clinical impacts were mostly for Hba1C, with some on BP and blood lipids
- Health impacts included mental health and BMI
- Patient expertise manifested in self-efficacy and greater knowledge
- System impacts were mostly seen as lower hospital admissions, with some evidence for cost effectiveness
Types of personalised care interventions
Our research identified three broad categories of personalized diabetic care intervention: telehealth, education-based, and peer support.
Telehealth interventions include self-management through remote communication (Hanlon et al, 2017), web-based programs (Hadjiconstantinou et al, 2016), interactive digital interventions (McLean et al, 2016), digital tools for children with type 1 diabetes (Knox et al, 2019), smartphone technologies for type 2 diabetes (Wu et al, 2018), wearables for monitoring (Mattison et al, 2022), nurse-led telerehabilitation (Lee et al, 2022), and computer-based self-management (Pal et al, 2013).
Education-based interventions aim to enhance diabetes management with tailored support, such as via the teach-back method (Dinh et al, 2016), culturally tailored diabetes education (Nam et al, 2012; Ricci-Cabello et al, 2014), individual face-to-face patient education for diabetes management (Duke et al, 2019), and as part of self-management for patients with severe mental illness (Mcbain et al, 2016).
Peer support programs were studied by Li Qi et al. (2017) and Elnaggar et al. (2018), focusing on structured education and social media-based interventions. Empowerment-based approaches, such as those by Chen et al. (2021) and Mogueo et al. (2016) targeted lifestyle modifications, self-management support, psychological interventions, and equipping patients with diabetes management skills.
Intervention effectiveness
Telehealth: Mobile phone interventions reduced HbA1c values by a mean of 0.5% over 6 months (Hanlon et al., 2017). Web-based interventions decreased feelings of depression and distress (Hadjiconstantinou et al., 2016). Interactive digital interventions significantly reduced systolic blood pressure (SBP) with a weighted mean difference (WMD) of -3.74mmHg (95% CI: -2.19 to -2.58; McLean et al., 2016). Smartphone technologies significantly reduced HbA1c levels with a pooled weighted mean difference of -0.51% (95% CI: -0.71% to -0.30%; p < 0.001; Wu et al., 2018). Nurse-led telehealth interventions improved systolic blood pressure, quality of life, self-efficacy, and overall depression levels (Wong et al., 2022; Lee et al., 2022). A wearable insole intervention reduced diabetic foot ulcer occurrence by 86% at 18-month follow-up (Mattison et al., 2022).
Education-based: Patient education interventions were found to increase medical adherence by 20% and knowledge scores in diabetes (Negarandeh, 2011; Swavely 2013). Culturally tailored diabetes educational interventions improved glycaemic control with a pooled effect size (ES) of -0.29 (95% CI: -0.46 to -0.13; Nam et al.). Ricci-Cabelo et al. reported that 59% of studies observed significant improvements in HbA1c and 57% of studies observed improvements in fasting blood sugar levels.
Peer Support: Pharmacist-led medication therapy resulted in a 52.1% reduction in hospital admissions (Mogueo et al., 2020). Empowerment-based interventions, when compared to routine care, were associated with reduced glycated haemoglobin levels, increased diabetes empowerment scores, and increased diabetes knowledge scores (Chen et al., 2021) with peer support interventions resulting in a pooled mean difference of -0.57% for HbA1c.
(Note: Summary table detailing the more significant impacts of personalised care can be found in Appendix 4).
Mediating Factors – Telehealth (Diabetes)
The following factors have been identified as impacting the effectiveness of their respective interventions.
Self-monitoring: In McLean, G. et al. (2016), it was found that self-monitoring increased the effectiveness of telehealth/decision support interventions (-4.02, 95% CI -2.93 to -5.12) compared to no self-monitoring (-0.88, 95% CI 0.05 to -1.80). In Pal, K. et al. (2013), it was also found that prompt self-monitoring of behavioural outcomes and providing feedback on performance were associated with more positive outcomes.
Duration of the intervention: In Pal, K. et al. (2013), they found that shorter studies with short-term follow-up appeared to have a larger effect size for HbA1c. In Hadjiconstantinou, M. et al. (2016), they discovered that the interventions that ran between 2 and 6 months showed significant improvement in distress or depression.
Age and duration of diagnosis: Wu, I.X. et al. (2018) found that a subgroup of T2DM patients (disease duration <8.5 years) appeared to benefit more from Smartphone Technology interventions. In Wu, I.X. et al. (2018), it was also found that Smartphone Technology interventions may provide a similar benefit to both younger and older T2DM patients.
Intervention delivery: In Hadjiconstantinou, M. et al. (2016), the majority of studies that provided professional support showed more promising results than those providing non-professional support. Whilst Pal, K. et al. (2013), found that interventions delivered via mobile phones performed better than interventions delivered at home.
Mediating Factors – Peer Support (Diabetes)
Baseline HbA1c levels: Qi, L. et al. (2015) found that the reduction in HbA1c levels was greater among patients with a baseline HbA1c level ≥ 8.5% and between 7.5 ~ 8.5%, compared to patients with a baseline HbA1c level < 7.5%. This suggests that peer support interventions may be more effective for patients with poor glycaemic control than for those with better glycaemic control.
Intervention structure: The same study reported that patients provided with individual intervention responded by a greater reduction in HbA1c level than patients provided with group session education or a combination of group and individual education. This suggests that individual peer support interventions may be more effective in reducing HbA1c levels.
Frequency of contact: Qi, L. et al. (2015) found that programs with a high or moderate frequency of contact reported a significant reduction in HbA1c levels compared with usual care, while programs with a low frequency of contact showed no significant reduction in HbA1c levels. This suggests that the frequency of contact is a key feature of the effectiveness of peer support interventions, and that only moderate or intensive interventions should be implemented.
Mode of delivery: There was no significant difference between the impact of community health workers or peer coaches as peer supporters. This suggests that both modes of delivery could be potential peer supporters based on different settings and populations.
Mediating Factors – Patient Education (Diabetes)
Health Literacy: People with low health literacy generally achieved greater disease-specific knowledge gains than those with high health literacy, indicating that the level of health literacy is a mediating factor in patient education effectiveness (Dinh et al., 2016).
Setting: Clinic or hospital-based diabetes educational centre settings were found to be more effective in reducing HbA1c levels compared to community-based settings, though this difference was not statistically significant (Nam, S. et al. 2012).
Baseline HbA1c level: This was found to affect the effectiveness of culturally tailored interventions, with the intervention being more effective for those with baseline HbA1c level equal to or less than 8.5% (Nam, S. et al. 2012).
Face-to-face patient education: This was found to be more effective in improving glycaemic control than telecommunication-based formats, although telecommunication programs have the potential to improve attrition rates (Ricci-Cabello et al, 2014).
Peer providers: Their involvement produced better results, as they provide a more credible source of information, empower those involved, and reinforce learning through ongoing contact (Ricci-Cabello et al, 2014).
Intervention Complexity: Less complex interventions led to greater improvements in glycaemic control (Ricci-Cabello et al, 2014).
Backed by theory: Theory-based interventions were found to produce more effects than non-theory-based interventions (Chen, Y. et al. 2021).
MSK
MSK synthesis: 8 papers showing positive effects
Variability in the papers
- 6 x Systematic Review
- 2 x meta-analysis
- 2 x <18
- 6 x adult
- variety of conditions, including osteoporosis, arthritis and lower back pain
- participant numbers ranged from 665 to 8539
- number of included studies ranged from 7 to 46
- variety of settings
- Papers mostly looked at SSM, with one for SDM
- use of numerous interventions
- 3 x self-management education
- 3 x technology enabled
- 1 x decision aids
- 1 x reflection
Areas of commonality
- Multi-study papers
- Telehealth (including = positive impacts on patient self-management expertise and physical health/ activity levels as well as level of pain
- SSM interventions broadly positive outcomes
Positive outcomes across two areas: pain (clinical) and wellbeing (such as physical/ mental health, satisfaction)
- Little support for assertions with regard to healthcare utilisation
- More research is needed, particularly of high quality
- Booster sessions generally showed no effect
Types of personalised care interventions
Shared decision-making: Coylewright et al. (2014) focused on using Decision Aids to facilitate shared decision-making between clinicians and patients.
Self-management interventions: Carnes et al. (2012), Donnelly et al. (2020), and Kroon et al. (2014) examined various self-management interventions for chronic musculoskeletal pain, rheumatoid arthritis, and osteoarthritis, respectively.
eHealth and mobile health technologies: Butler et al. (2021) and Mattison et al. (2022) assessed the effectiveness of eHealth, mobile health, and wearable interventions in supporting specific patient populations.
Multidisciplinary approaches and structured programs: Buzasi et al. (2021) and Safari et al. (2019) investigated the use of single modality and multidisciplinary approaches for pain management, combining various treatments and theories.
Intervention effectiveness
In Coylewright, M (2014), decision support tools were found to improve patients’ general knowledge, as participants who received care with decision aids had greater gains in knowledge compared to those who received usual care (62% vs 45%, p<0.0001). Patients who used decision aids also had a better understanding of their personalized risk (50% vs 20%, p<0.0001), and experienced lower decisional conflict (13 points vs 18 points). Clinicians also encouraged patient empowerment significantly more often when using decision support (39 vs 21).
Telehealth interventions were also found to be effective in reducing school absenteeism (43% to 14%) in one study (Butler et al, 2021), and in reducing pain intensity (1.73-point reduction on 1-10 scale) in another study (Butler et al, 2021). A telehealth intervention using FitBit (Mattison, G.,et al, 2022) showed an increase of 183.1 min/week in walking time.
Self-management education programs, including booster sessions (Buzasi, E. et al, 2021) were found to be effective in reducing pain in patients with chronic pain conditions. In (Buzasi, E. et al, 2021), there was a significant reduction in pain catastrophizing (SMD 20.42; 95% CI), while in Kroon, F et al (2019), the SMD between groups was ‐0.26 (95% CI ‐0.44 to ‐0.09), with a mean reduction of 0.8 points on the VAS Scale.
Finally, a digital-based structured self-management program was found to be effective in reducing pain (5.7% reduction) and improving physical function (5.07% improvement) in Safari, R.et al 2019. The same study showed that digital-based structured self-management programs were more effective than health education conditions (SMD 0.26; 95% CI).
(Note: Summary table detailing the more significant impacts of personalised care can be found in Appendix 4).
Mediating factors – MSK
Reporting frequency: A systematic review and meta-analysis of eHealth and mobile health interventions supporting children and young people living with Juvenile Idiopathic Arthritis found that adherence rates were higher when pain was reported once a week compared with when pain was reported once a day or twice a day or as and when pain was experienced. However, there were no significant differences in pain interference scores because of reporting frequency, and the children and young people reported that they preferred once a day or as and when reporting schedules (Butler et al., 2021).
Seasonal fluctuations: A study found a seasonal intervention effect when comparing a winter intervention group to a summer intervention group for physical activity promotion. For the winter group, a 24-minute reduction in rest was recorded using an accelerometer, and this result was significant (Butler et al., 2021).
Intervention components: There may be no one-size-fits-all intervention, or there may be a need for a combination of interventions. For example, a pain self-management application combined two interventions (real-time pain monitoring and self-management) and then compared this combination to standalone pain monitoring. This combination demonstrated a greater decrease in pain intensity scores, and although this finding was not significant, the inclusion of self-management programs could be clinically beneficial. Our research found evidence in the benefit of having a psychological component across most outcomes in the short and medium-term. In the short term, self-management was favoured for most outcomes irrespective of the presence of a pain education component, but in the medium-term, there was more evidence in favour (Carnes et al., 2012).
Setting: A review of effective delivery styles and content for self-management interventions for chronic musculoskeletal pain found that there was evidence of benefit for courses held in medical settings for all outcomes in the short term. Courses in community settings showed statistically significant beneficial effects for self-efficacy across all time points. Occupational settings were too rarely reported to make inferences about their effect (Carnes et al., 2012).
Mode of delivery: The number of disciplines involved in intervention delivery and the method of delivery (remote vs face to face) influenced the effect of self-management booster sessions. The different intensities of the main intervention did not influence physical function and pain-related disability, and treatment type and method of delivery of boosters did not affect physical function.
COPD
COPD synthesis: 4 papers with positive effects
Variability in the papers
- 3 x Systematic Review
- 3 x meta-analysis
- 2 x <18
- 6 x >18
- All COPD patients, one adding asthma
- participant numbers ranged from 439 to 6008
- number of included studies ranged from 8 to 27
- variety of settings
- use of numerous interventions
- multiple impacts across different categories
Areas of commonality
- All papers focussed on SSM
- All papers explored COPD patients
Types of personalised care interventions
Several interventions have been studied in relation to chronic obstructive pulmonary disease (COPD). Long et al. (2019) investigated health coaching interventions, which included goal setting, motivational interviewing, and COPD-related health education. Song et al. (2021) conducted a systematic review and meta-analysis of blended self-management interventions that combined eHealth with face-to-face interventions. Collins et al. (2012) studied nutritional support, mainly in the form of personalised supplementation. Schrijver et al. (2022) reviewed self-management interventions for people living with COPD, which included smoking cessation, self-recognition of exacerbations, use of an exacerbation action plan, home-based exercise or physical activity, diet and medication intake, and coping with breathlessness. Lenferink et al. (2017) conducted a systematic review and meta-analysis of blended self-management interventions that combined eHealth with face-to-face interventions, specifically mobile application and individual face-to-face intervention or internet-assisted intervention and group face-to-face interaction. McCabe et al. (2017) studied self-management interventions, which typically involve an iterative process of setting goals, monitoring progress, and making adjustments based on feedback, often utilizing behavioural change techniques such as goal setting and problem solving.
Intervention effectiveness
Long et al., 2019 examined the impact of an intervention on hospital admissions, reporting a significant reduction in hospital admissions (OR = 0.46; 95% CI: 0.31, 0.69; p = 0.0001), based on five studies. Song et al., 2021 evaluated a blended self-management intervention and found a reduction in exacerbation frequency with a relative risk of 0.38 (95% CI 0.26-0.56), as well as a significant reduction in BMI with a mean difference of 0.81 (95% CI 0.25-1.34) and an improvement in quality of life with a standardized mean difference of 0.81.
Collins et al (2012) investigated the impact of nutritional support and found significantly greater increases in mean total protein and energy intakes, with a mean difference of 1.94 ± 0.26 kg (p<0.001). Schrijver, J et al (2022) evaluated self-management interventions and found a 2.86-point lower score on the St. George’s Respiratory Questionnaire adjusted total score, indicating significantly better health-related quality of life (95% CI 4.87 lower vs 0.85 lower). The same study also reported a lower risk of emergency department visits with a hazard ratio of -0.52 (95% CI), as well as an improvement in exercise capability with a mean difference of 45.14 meters in walking (95% CI 9.16 to 81.13). Additionally, self-management interventions that included action plans and smoking cessation programs were found to contribute to significant improvements in health-related quality of life, with a mean difference from usual care of -2.69 points (95% CI -4.49 to -0.90).
Lenferink et al., (2017) evaluated the impact of self-management education on activity levels, reporting significant improvements in exercise capacity with a pooled mean difference of 45.14 meters in the six-minute walking test (95% CI). Additionally, self-management interventions, including exacerbation action plans with smoking cessation programs, were found to significantly improve health-related quality of life, with a mean difference from usual care of -2.69 points (95% CI -4.49 to -0.90).
In summary, these studies provide evidence supporting the effectiveness of various interventions, including decision support, telehealth, self-management education, and nutritional support, for improving outcomes such as hospital admissions, exacerbation frequency, BMI, quality of life, and exercise capacity.
Mediating Factors – COPD
Frequency and difficulty: For health coaching interventions (Long et al., 2019), the frequency and number of coaching sessions, the difficulty of goals, and baseline HRQoL scores can impact effectiveness.
Intervention components: For self-management interventions (Schrijver, J, 2022), factors such as home-based exercise, COPD action plans, smoking cessation, and coping with breathlessness can impact effectiveness. Smoking cessation and digital components might not significantly affect HRQoL, while medium-term interventions show better results in HRQoL improvement.
Mode of delivery: Smart technology interventions proved more effective than face-to-face or written support, improving symptoms and impacts (McCabe, C 2017)). The frequency of assessment also plays a role in the intervention’s effectiveness, with significant HRQoL improvements observed at four weeks, four months, and six months.
Overarching Themes
Given the breadth of Personalised Care and the populations that studies have focussed on, we undertook a brief thematic extraction across all findings.
- Quality of the reviews assessed was variable
- Most studies focus on SSM, across all conditions, with little on the other areas.
- impact of PC interventions can be see in 5 areas:
- patient expertise in managing their condition. This includes understanding of their condition, greater efficacy in management, and empowerment
- Clinical. Measured using standard clinical outcomes. Evidence varies in strength.
- General health, both physical and mental. The review of NICE Guidelines strongly supported this theme.
- System. Clear evidence that PC interventions reduce hospital admissions, at least for diabetes and COPD.
- Quality of life. Factors beyond health that improve the patient’s quality of life
Discussion
The NHS has made a commitment to Personalised Care. There is some evidence to support the increase in deployment of PC interventions, and this review adds to that, for these three specific condition areas.
The original brief for this review asked us to explore, for PC in diabetes, MSK and COPD has:
- A strong evidence base
The evidence in each condition area varies. For MSK, our research returned no evidence with regard to system impact via lower admissions, healthcare usage and the like. The impacts are secondary, via lower levels of pain and higher levels of patient expertise in managing their condition. We might speculate and extrapolate that this will lead ultimately to a reduction in system pressures, but this will manifest beyond the timescale of most studies. For diabetes, there is some of evidence to support an improvement in clinical outcomes, and indeed for a reduction in system pressures mostly through lower levels of hospitalisation. The former can be implied to impact system utilisation indirectly, through less interaction with primary care, although this has not been explicitly examined. For COPD, the evidence for impact on healthcare utilisation is well founded.
- A key role in improving health and care
What is clear from this review is that PC interventions impact patients’ lives in many positive ways. They can help them to better understand and manage their condition, leading to a profound improvement in their lived experience. It can help them to feel more supported and empowered, better able to cope with what might be a debilitating long-term condition. It can help them to feel more in control of their condition, and therefore more able to participate in society and the economy. These indirect impacts are laudable, but the current financial pressures require initiatives to justify their existence over and above care as usual, through measurable system impacts. Only two of the condition areas show evidence for this.
- Aligned to a high priority area for the NHS
Alignment of research evidence with areas of high priority for policy-makers can be uneven. Research will take place where funding is available, and funding will follow research priorities, which may or may not align with policy priorities. In this case, there is good evidence that SSM and to a lesser extent SDM and SP are effective in impacting healthcare utilisation. There is an argument for a deep dive into the variation in activity around SSM and in fact all PC activity to reduce variation and level up areas with a deficit. In addition, there may be value in exploring the relationship between deprivation and other wider determinants of health, in areas where PC may have a higher impact, due to the higher prevalence of social deficits.
- Can demonstrate high impact to current priorities
The primary theme of this set of literature is that SSM works to reduce hospital admissions in diabetes and COPD. The evidence is not there for MSK, partly perhaps because this is a condition area that is less likely to lead to hospitalisation when exacerbated, and partly because the studies have not been carried out. More broadly, the quality literature has focussed almost exclusively on SSM, with very little looking at the other PC interventions. More research is required to explore the impact of PCSPs and PHBs, as well as social prescribing. Another notable gap in the literature is around healthcare utilisation. Most of the evidence is focussed on secondary care. While this is laudable, there may be significant undetected impacts in primary care, which is where most management of long-term conditions takes place. If PC can prevent a GP visit, then the impact across the system could be substantial.
Limitations
The primary limitation to this review is the necessarily truncated nature of the methodology. Due to the high number of papers found, we restricted the review to the most probative, which would at the same time cover the broadest landscape, being systematic reviews and meta-analyses. The evidence we have analysed and presented may not represent the totality, but is robust and verifiable.
There are four important areas that the literature does not consider: the impact of using more than one of the PC components as a planned flow of intervention, patients with multiple morbidities, the impact on Primary Care, and the effect on patients via the workforce.
The different components and staff members in PC are not designed to operate in isolation. The person-centred approach is designed to explore the needs of that individual, and tailor care to those needs. There may be, therefore, a role for more than one of the components, which together may have the positive impact on their care, experience and usage of the health system. The infographic below (from the NHS Universal Personalised Care report) illustrates this well.
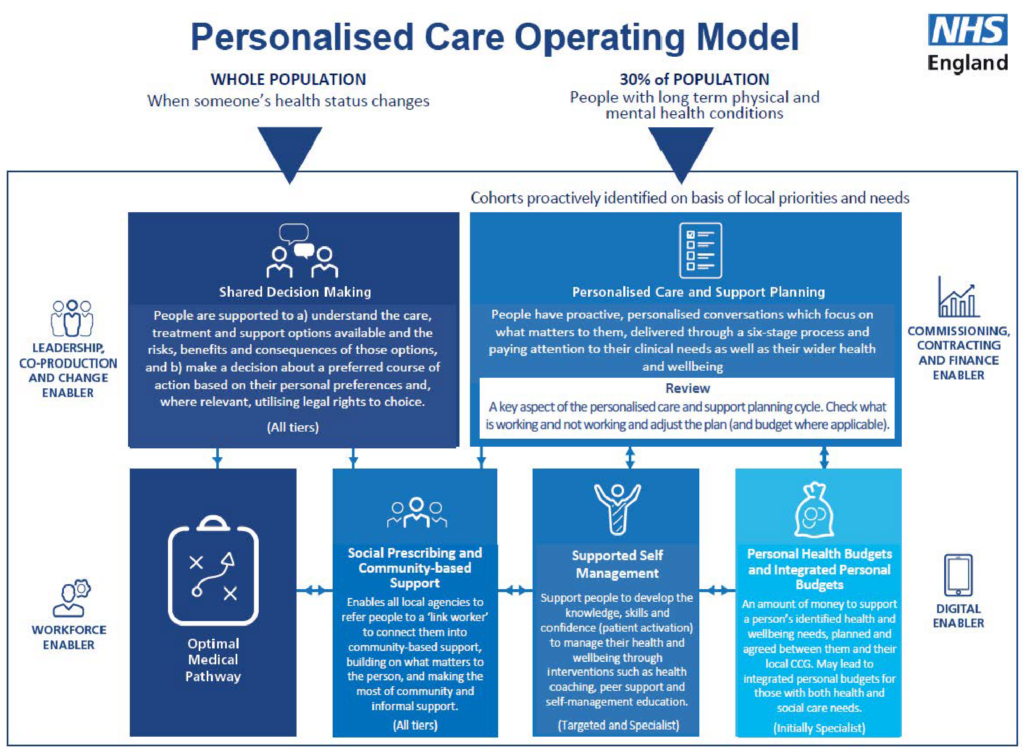
In many cases, the implementation of PC interventions will be iterative, over the course of a patient’s illness, cumulative, or possible in series. Current research does not capture the impact for these patients.
Increasingly, patients are presenting to care with more than one condition or illness, often these being long-term conditions. As our population ages, this issue will become more challenging. This review, necessarily, focussed on the three condition areas as described, diabetes, MSK and COPD, and therefore did not specifically seek research that used comorbid patients as the study populations.
None of the research considered within this review looked at the impact of PC interventions in Primary Care. The evidence shows, via a variety of effects, that there is clear impact on patients’ health and wellbeing, both physical and mental. Better managed health would arguably lead to less interaction with primary care, where the majority of management for patients with long-term conditions takes place. Given the pressures currently affecting primary care, this is an important gap in our knowledge.
The literature has yet to consider the impact on staff of personalised care interventions. There is an argument that personalised care may bring benefit to staff working with those patients; in the current environment of staffing pressures in the NHS, anything that may improve morale should be explored (Dave Pearson’s story, page 36, https://www.england.nhs.uk/wp-content/uploads/2019/01/universal-personalised-care.pdf)
Finally, this review included only the three prescribed condition areas. Further reviews could look at other long-term conditions.
Conclusions & recommendations
- In some forms for some conditions, the evidence supports the assertion that PC leads to lower healthcare utilisation. The evidence is strongest for SSM in COPD. Increasing this will have a positive impact on use of secondary care.
- There is cross-condition support for the positive impact that PC has on patient health, wellbeing and clinical outcomes
- Deep dive analyses would be instructive to identify areas of variation and relationships of PC interventions to deprivation and other wider determinants of health
- More research is required to understand more about the other components of PC and their impact, as well as more exploration of how the indirect impacts might reduce utilisation in the longer term
- More evidence is required to explore the person-centric impact of PC, not just one of the components or its interventions in isolation of the others
- More information is needed about the impact of PC interventions on utilisation in primary care
- An evidence review could look at co-morbid patients, and the impact that PC interventions have for them
- Further exploration could be made of the impact on staff working in and around PC
References
Process and methodology
Almeida MO, Yamato TP, Parreira PDCS, Costa LOP, Kamper S, Saragiotto BT. Overall confidence in the results of systematic reviews on exercise therapy for chronic low back pain: a cross-sectional analysis using the Assessing the Methodological Quality of Systematic Reviews (AMSTAR) 2 tool. Braz J Phys Ther. 2020 Mar-Apr;24(2):103-117. doi: 10.1016/j.bjpt.2019.04.004. Epub 2019 May 8. PMID: 31113734; PMCID: PMC7082685.
British Lung Foundation (2016). Estimating the economic burden of respiratory illness in the UK. https://cdn.shopify.com/s/files/1/0221/4446/files/PC-1601_-_Economic_burden_report_FINAL_8cdaba2a-589a-4a49-bd14-f45d66167795.pdf?1309501094450848169&_ga=2.212108820.1040159959.1677169561-312710585.1677169561
Department of Health (2012). COPD Commissioning Toolkit. https://assets.publishing.service.gov.uk/government/uploads/system/uploads/attachment_data/file/212876/chronic-obstructive-pulmonary-disease-COPD-commissioning-toolkit.pdf
Dixon-Woods M, Cavers D, Agarwal S, Annandale E, Arthur A, Harvey J, Hsu R, Katbamna S, Olson R, Smith L, Riley R, Sutton AJ. Conducting a critical interpretive synthesis of the literature on access to healthcare by vulnerable groups. BMC Med Res Methodol. 2006;6:3. doi: 10.1186/1471-2288-6-35.
Higgins JPT, Green S. Cochrane Handbook for Systematic Reviews of Interventions, Version 5.1.0. The Cochrane Collaboration. 2013
Hsieh H, Shannon S (2005). Three approaches to qualitative content analysis. Qualitative Health Research, 15, 1277 – 1287.
Methley AM, Campbell S, Chew-Graham C, McNally R, Cheraghi-Sohi S. PICO, PICOS and SPIDER: a comparison study of specificity and sensitivity in three search tools for qualitative systematic reviews. BMC Health Serv Res. 2014
National Voices (2014) Supporting self-management: summarising the evidence from systematic reviews. https://www.nationalvoices.org.uk/sites/default/files/public/publications/supporting_self-management.pdf
Nesta (2016). At the Heart of Health: realising the value of people and communities. https://media.nesta.org.uk/documents/at_the_heart_of_health_-_realising_the_value_of_people_and_communities.pdf
Noyes J. Never mind the qualitative feel the depth! The evolving role of qualitative research in Cochrane intervention reviews. J Res Nurs. 2010;15:525–534.
NHS (2019). The NHS long term plan. https://www.longtermplan.nhs.uk/
NHS (2019) (2). Universal Personalised Care. https://www.england.nhs.uk/wp-content/uploads/2019/01/universal-personalised-care.pdf
NICE (2022) Developing NICE Guidelines: the manual. Updated January 2022. https://www.nice.org.uk/process/pmg20/chapter/introduction
Page MJ, McKenzie JE, Bossuyt PM, Boutron I, Hoffmann TC, Mulrow CD, et al. The PRISMA 2020 statement: an updated guideline for reporting systematic reviews. BMJ 2021;372:n71. doi: 10.1136/bmj.n71.
Pieper D, Antoine SL, Mathes T, Neugebauer EA, Eikermann M. Systematic review finds overlapping reviews were not mentioned in every other overview. J Clin Epidemiol. 2014 Apr;67(4):368-75. doi: 10.1016/j.jclinepi.2013.11.007. PMID: 24581293.
Popay, J., Roberts, H.M., Sowden, A.J., Petticrew, M., Arai, L., Rodgers, M., & Britten, N. (2006). Guidance on the conduct of narrative synthesis in systematic Reviews. A Product from the ESRC Methods Programme. Version 1.
Ricci-Cabello, I., Ruiz-Pérez, I., Rojas-García, A. et al. Characteristics and effectiveness of diabetes self-management educational programs targeted to racial/ethnic minority groups: a systematic review, meta-analysis and meta-regression. BMC Endocr Disord 14, 60 (2014). https://doi.org/10.1186/1472-6823-14-60
Shea B J, Reeves B C, Wells G, Thuku M, Hamel C, Moran J et al. AMSTAR 2: a critical appraisal tool for systematic reviews that include randomised or non-randomised studies of healthcare interventions, or both BMJ 2017; 358 :j4008 doi:10.1136/bmj.j4008
Smith, V., Devane, D., Begley, C.M. et al. Methodology in conducting a systematic review of systematic reviews of healthcare interventions. BMC Med Res Methodol 11, 15 (2011). https://doi.org/10.1186/1471-2288-11-15
Suarez-Almazor ME, Belseck E, Homik J, Dorgan M, Ramos-Remus C. Identifying clinical trials in the medical literature with electronic databases: MEDLINE alone is not enough. Control Clin Trials. 2000 Oct;21(5):476-87. doi: 10.1016/s0197-2456(00)00067-2. PMID: 11018564.
Taylor S, Pinnock H, Epiphanou E, Pearce G, Parke H, Schwappach A, et al. A rapid synthesis of the evidence on interventions supporting self-management for people with long-term conditions (PRISMS Practical systematic Review of Self-Management Support for long-term conditions). Health Serv Deliv Res 2014;2(53)
Watt, A., Cameron, A., Sturm, L., et al. (2008) Rapid reviews versus full systematic reviews: an inventory of current methods and practice in health technology assessment. International Journal of Technology Assessment in Health Care, 24 (2), 133–139.
Weiss CH. Evaluation: methods for studying programs and policies. New Jersey: Prentice-Hall, 1998.
Diabetes
Dinh, H.T.T., Bonner, A., Clark, R., Ramsbotham, J. and Hines, S. (2016c). The effectiveness of the teach-back method on adherence and self-management in health education for people with chronic disease: a systematic review. The JBI Database of Systematic Reviews and Implementation Reports, [online] 14(1), p.210. doi:https://doi.org/10.11124/jbisrir-2016-2296.
Qi, L., Liu, Q., Qi, X. et al. Effectiveness of peer support for improving glycaemic control in patients with type 2 diabetes: a meta-analysis of randomized controlled trials. BMC Public Health 15, 471 (2015). https://doi.org/10.1186/s12889-015-1798-y
Nam, Soohyun PhD, RN, NP; Janson, Susan L. DNSc, RN, FAAN; Stotts, Nancy A. EdD, RN, FAAN; Chesla, Catherine DNSc, RN, FAAN; Kroon, Lisa PharmD, CDE. Effect of Culturally Tailored Diabetes Education in Ethnic Minorities With Type 2 Diabetes: A Meta-analysis. The Journal of Cardiovascular Nursing 27(6):p 505-518, November/December 2012. | DOI: 10.1097/JCN.0b013e31822375a5
Hanlon P, Daines L, Campbell C, McKinstry B, Weller D, Pinnock HTelehealth Interventions to Support Self-Management of Long-Term Conditions: A Systematic Metareview of Diabetes, Heart Failure, Asthma, Chronic Obstructive Pulmonary Disease, and Cancer J Med Internet Res 2017;19(5):e172 URL: http://www.jmir.org/2017/5/e172/ doi: 10.2196/jmir.6688 PMID: 28526671″
Hadjiconstantinou M, Byrne J, Bodicoat D, Robertson N, Eborall H, Khunti K, Davies M, ‘Do Web-Based Interventions Improve Well-Being in Type 2 Diabetes? A Systematic Review and Meta-Analysis’, J Med Internet Res 2016;18(10):e270, URL: https://www.jmir.org/2016/10/e270, DOI: 10.2196/jmir.5991
McLean, Gary; Band, Rebecca; Saunderson, Kathryn; Hanlon, Peter; Murray, Elizabeth; Little, Paul; McManus, Richard J.; Yardley, Lucy; Mair, Frances S. on behalf of the DIPSS co-investigators. Digital interventions to promote self-management in adults with hypertension systematic review and meta-analysis. Journal of Hypertension 34(4):p 600-612, April 2016. | DOI: 10.1097/HJH.0000000000000859
Knox, E.C.L., Quirk, H., Glazebrook, C. et al. Impact of technology-based interventions for children and young people with type 1 diabetes on key diabetes self-management behaviours and prerequisites: a systematic review. BMC Endocr Disord 19, 7 (2019). https://doi.org/10.1186/s12902-018-0331-6
Coronado-Vázquez, V., Canet-Fajas, C., Delgado-Marroquín, M.T., Magallón-Botaya, R., Romero-Martín, M. and Gómez-Salgado, J. (2020). Interventions to facilitate shared decision-making using decision aids with patients in Primary Health Care. Medicine, 99(32), p.e21389. doi:https://doi.org/10.1097/md.0000000000021389.
Wu, I.X.Y., Kee, J.C.Y., Threapleton, D.E., Ma, R.C.W., Lam, V.C.K., Lee, E.K.P., Wong, S.Y.S. and Chung, V.C.H. (2018). Effectiveness of smartphone technologies on glycaemic control in patients with type 2 diabetes: systematic review with meta-analysis of 17 trials. Obesity Reviews, 19(6), pp.825–838. doi:https://doi.org/10.1111/obr.12669.
Elnaggar, A., Park, V.T., Lee, S.J., Bender, M., Siegmund, L.A. and Park, L.G. (2020). Patients’ Use of Social Media for Diabetes Self-Care: Systematic Review. Journal of Medical Internet Research, [online] 22(4), p.e14209. doi:https://doi.org/10.2196/14209.
Wong, A.K.C., Bayuo, J., Wong, F.K.Y., Yuen, W.S., Lee, A.Y.L., Chang, P.K. and Lai, J.T.C. (2022). Effects of a Nurse-Led Telehealth Self-care Promotion Program on the Quality of Life of Community-Dwelling Older Adults: Systematic Review and Meta-analysis. Journal of Medical Internet Research, [online] 24(3), p.e31912. doi:https://doi.org/10.2196/31912.
Mattison, G., Canfell, O., Forrester, D., Dobbins, C., Smith, D., Töyräs, J. and Sullivan, C. (2022c). The Influence of Wearables on Health Care Outcomes in Chronic Disease: Systematic Review. Journal of Medical Internet Research, 24(7), p.e36690. doi:https://doi.org/10.2196/36690.
Mogueo, A., Oga‐Omenka, C., Hatem, M. and Kuate Defo, B. (2020). Effectiveness of interventions based on patient empowerment in the control of type 2 diabetes in sub‐Saharan Africa: A review of randomized controlled trials. Endocrinology, Diabetes & Metabolism, 4(1). doi:https://doi.org/10.1002/edm2.174.
Lee, A.Y.L., Wong, A.K.C., Hung, T.T.M., Yan, J. and Yang, S. (2022). Nurse-led Telehealth Intervention for Rehabilitation (Telerehabilitation) Among Community-Dwelling Patients With Chronic Diseases: Systematic Review and Meta-analysis. Journal of Medical Internet Research, 24(11), p.e40364. doi:https://doi.org/10.2196/40364.
Ricci-Cabello, I., Ruiz-Pérez, I., Rojas-García, A. et al. Characteristics and effectiveness of diabetes self-management educational programs targeted to racial/ethnic minority groups: a systematic review, meta-analysis and meta-regression. BMC Endocr Disord 14, 60 (2014). https://doi.org/10.1186/1472-6823-14-60
Chen, Y., Tian, Y., Sun, X., Wang, B. and Huang, X. (2021). Effectiveness of empowerment-based intervention on HbA1c and self-efficacy among cases with type 2 diabetes mellitus. Medicine, 100(38), p.e27353. doi:https://doi.org/10.1097/md.0000000000027353.
Pal, K., Eastwood, S.V., Michie, S., Farmer, A.J., Barnard, M.L., Peacock, R., Wood, B., Inniss, J.D. and Murray, E. (2013). Computer-based diabetes self-management interventions for adults with type 2 diabetes mellitus. The Cochrane Database of Systematic Reviews, [online] (3), p.CD008776. doi:https://doi.org/10.1002/14651858.CD008776.pub2.
Duke, S.-A.S., Colagiuri, S. and Colagiuri, R. (2009). Individual patient education for people with type 2 diabetes mellitus. Cochrane Database of Systematic Reviews. [online] doi:https://doi.org/10.1002/14651858.cd005268.pub2.
McBain, H., Mulligan, K., Haddad, M., Flood, C., Jones, J. and Simpson, A. (2016). Self management interventions for type 2 diabetes in adult people with severe mental illness. Cochrane Database of Systematic Reviews. doi:https://doi.org/10.1002/14651858.cd011361.pub2.
MSK
Coylewright, M., Branda, M., Inselman, J.W., Shah, N., Hess, E., LeBlanc, A., Montori, V.M. and Ting, H.H. (2014). Impact of Sociodemographic Patient Characteristics on the Efficacy of Decision Aids. Circulation: Cardiovascular Quality and Outcomes, 7(3), pp.360–367. doi:https://doi.org/10.1161/hcq.0000000000000006.
Carnes, D., Homer, K.E., Miles, C.L., Pincus, T., Underwood, M., Rahman, A. and Taylor, S.J.C. (2012). Effective Delivery Styles and Content for Self-management Interventions for Chronic Musculoskeletal Pain. The Clinical Journal oF Pain, 28(4), pp.344–354. doi:https://doi.org/10.1097/ajp.0b013e31822ed2f3.
Butler, S., Sculley, D., Santos, D., Fellas, A., Gironès, X., Singh-Grewal, D. and Coda, A. (2021). Effectiveness of eHealth and mobile Health interventions supporting children and young people living with Juvenile Idiopathic Arthritis: systematic review and meta-analysis (Preprint). Journal of Medical Internet Research. doi:https://doi.org/10.2196/30457.
Mattison, G., Canfell, O., Forrester, D., Dobbins, C., Smith, D., Töyräs, J. and Sullivan, C. (2022b). The Influence of Wearables on Health Care Outcomes in Chronic Disease: Systematic Review. Journal of Medical Internet Research, 24(7), p.e36690. doi:https://doi.org/10.2196/36690.
Buzasi, E., Kurakata, H., Gandhi, A., Birch, H.L., Zarnegar, R. and Best, L. (2021). The effects of booster sessions on self-management interventions for chronic musculoskeletal pain. Pain, Publish Ahead of Print. doi:https://doi.org/10.1097/j.pain.0000000000002302.
Safari, R., Jackson, J. and Sheffield, D. (2019). Digital-based self-management interventions for people with osteoarthritis: Systematic review with meta-analysis (Preprint). Journal of Medical Internet Research. doi:https://doi.org/10.2196/15365.
Donnelly, S., Manning, M., Mannan, H., Wilson, A.G. and Kroll, T. (2020). Renegotiating dimensions of the self: A systematic review and qualitative evidence synthesis of the lived experience of self‐managing rheumatoid arthritis. Health Expectations. doi:https://doi.org/10.1111/hex.13122.
Kroon, F.P., van der Burg, L.R., Buchbinder, R., Osborne, R.H., Johnston, R.V. and Pitt, V. (2014). Self-management education programmes for osteoarthritis. Cochrane Database of Systematic Reviews. doi:https://doi.org/10.1002/14651858.cd008963.pub2.
COPD
Long, H., Howells, K., Peters, S. and Blakemore, A. (2019b). Does health coaching improve health‐related quality of life and reduce hospital admissions in people with chronic obstructive pulmonary disease? A systematic review and meta‐analysis. British Journal of Health Psychology, 24(3). doi:https://doi.org/10.1111/bjhp.12366.
Song, X., Hallensleben, C., Zhang, W., Jiang, Z., Shen, H., Gobbens, R.J.J., Kleij, R.M.J.J.V.D., Chavannes, N.H. and Versluis, A. (2021). Blended Self-Management Interventions to Reduce Disease Burden in Patients With Chronic Obstructive Pulmonary Disease and Asthma: Systematic Review and Meta-analysis. Journal of Medical Internet Research, 23(3), p.e24602. doi:https://doi.org/10.2196/24602.
Collins, P.F., Stratton, R.J. and Elia, M. (2012). Nutritional support in chronic obstructive pulmonary disease: a systematic review and meta-analysis. The American Journal of Clinical Nutrition, 95(6), pp.1385–1395. doi:https://doi.org/10.3945/ajcn.111.023499.
Schrijver, J., Lenferink, A., Brusse-Keizer, M., Zwerink, M., van der Valk, P.D., van der Palen, J. and Effing, T.W. (2022). Self-management interventions for people with chronic obstructive pulmonary disease. Cochrane Database of Systematic Reviews, 2022(1). doi:https://doi.org/10.1002/14651858.cd002990.pub4.
Lenferink, A., Brusse-Keizer, M., van der Valk, P.D., Frith, P.A., Zwerink, M., Monninkhof, E.M., van der Palen, J. and Effing, T.W. (2017). Self-management interventions including action plans for exacerbations versus usual care in patients with chronic obstructive pulmonary disease. Cochrane Database of Systematic Reviews. doi:https://doi.org/10.1002/14651858.cd011682.pub2.
McCabe, C., McCann, M. and Brady, A.M. (2017). Computer and mobile technology interventions for self-management in chronic obstructive pulmonary disease. Cochrane Database of Systematic Reviews. doi:https://doi.org/10.1002/14651858.cd011425.pub2.
Appendices
Appendix 1: Original brief
Personalised Care is becoming ‘business as usual’ in the NHS, and is part of the NHS Long Term Plan – and indeed is more vital than ever in the context of the recovery of elective care (and indeed other demands) in the NHS in the wake of Covid-19.
In this context, the Personalised Care Group are seeking to commission a literature review of personalised care interventions in key, high impact, clinical specialist areas in order to give confidence to the system of the impact and efficacy of these interventions and encourage their further uptake.
Specific objectives
Establish 3 key high impact areas where felt personalised care has
- A strong evidence base
- A key role in improving health and care
- Aligned to a high priority area for the NHS
- Can demonstrate high impact to current priorities
Conduct and produce literature review of the evidence in these key areas.
Develop resource/products for each high impact area that can be used to influence decision making where personalised care makes its greatest impact.
These areas are to be Diabetes, MSK and acute respiratory disease (COPD).
Research questions
To de defined further throughout commission. Key overarching area includes:
What is the evidence based impact of personalised care in MSK, diabetes and respiratory care? Particularly looking at evidence aligned to
- Volume
- Needs
- Inequity
- And where personalised care interventions are mostly taking place
Personalised care is defined in the criteria below using the NHS England universal model for personalised care.
Inclusion criteria
i. Population, or participants and conditions of interest | Those who have MSK, diabetes, and COPD (3 key high impact areas) |
ii. Interventions | 5 components of the Universal model of Personalised Care Shared Decision Making Supported Self Management Social Prescribing Personalised Care and Support Planning Choice |
iii. Comparisons or control groups | No comparator |
iv. Outcomes of interest | Impacts on health utilisation (admissions, A&E, GP attendance etc) and impact on clinical outcomes, wellbeing outcomes, patient experience & safety. |
v. Setting | To be setting agnostic but more likely to take place in the community given the policy direction & investment to date and the primary but not only location of the workforce |
vi. Study designs | Systematic Reviews / Meta-analysis |
Exclusion criteria
Our work hasn’t included evidence that isn’t in English (check with them)
Exclude anything over 10 years old – but please highlight if most evidence is over 10 years old which would leave us with too little to analyse
Search methods
- Electronic databases
- Medline
- Embase
- Cochrane
Presentation of results
3 products – one for each high impact area of MSK, diabetes and acute respiratory diseases (COPD)
Appendix 2: Search strings
PICOS Element | Key words | Search terms |
---|---|---|
Patient or Population | MSK, diabetes, respiratory disease (Asthma/COPD etc). | Population (P): MSK, diabetes or COPD Embase specific subject headings: MSK – exp Musculoskeletal Diseases/ (this also covers: musculoskeletal complaint, musculoskeletal diseases, musculoskeletal disorder, musculoskeletal symptom) Diabetes – non insulin dependent diabetes mellitus/ (this is covers: adult onset diabetes, adult onset diabetes mellitus, diabetes mellitus type 2, diabetes mellitus type ii, diabetes mellitus, maturity diabetes mellitus, diabetes mellitus, non insulin dependent, diabetes mellitus, non−insulin−dependent, diabetes mellitus, type 2 [MeSH Descriptor], diabetes mellitus, type II, diabetes type 2, diabetes type II, diabetes, adult, dm 2, insulin independent diabetes, insulin independent diabetes mellitus, ketosis resistant diabetes mellitus, maturity onset diabetes, mellitus maturity onset diabetes mellitus, maturity onset diabetes of the young, diabetes mellitus, niddm, NIDDM (non insulin dependent diabetes mellitus), non insulin dependent diabetes, non−insulin−dependent diabetes mellitus, noninsulin dependent diabetes, noninsulin dependent diabetes mellitus, T2DM, type 2 diabetes, type 2 diabetes mellitus, type II diabetes, type II diabetes mellitus) – pregnancy diabetes mellitus/ (this also covers: diabetes mellitus gravidarum, diabetes, gestational, diabetes, pregnancy, gestational diabetes, gestational diabetes mellitus, pregnancy diabetes, pregnancy in diabetics – experimental diabetes mellitus/ (this also covers: diabetes mellitus, experimental, experimental diabetes, experimentally induced diabetes, experimentally induced diabetes mellitus) – insulin dependent diabetes mellitus/ (this also covers: brittle diabetes, brittle diabetes mellitus, diabetes mellitus type 1, diabetes mellitus type I, diabetes mellitus, brittle, diabetes mellitus, insulin dependent, diabetes mellitus, insulin−dependent, diabetes mellitus, juvenile onset, mellitus, diabetes mellitus, type 1, diabetes mellitus, type I, diabetes type 1, diabetes type I, diabetes, juvenile, dm 1, early onset diabetes mellitus, iddm, insulin dependent diabetes, insulin−dependent diabetes mellitus, juvenile diabetes, diabetes mellitus, juvenile onset diabetes, juvenile onset diabetes mellitus mellitus, ketoacidotic diabetes, labile diabetes mellitus, mckusick 22210, T1DM, type 1 diabetes, type 1 diabetes mellitus, type I diabetes, type I diabetes mellitus) COPD – chronic obstructive lung disease/ (this also covers: chronic airflow obstruction, chronic airway obstruction, chronic obstructive bronchopulmonary disease, chronic obstructive lung disorder, chronic obstructive pulmonary disease, chronic obstructive pulmonary disorder, chronic obstructive respiratory disease, chronic pulmonary obstructive disease, chronic pulmonary obstructive disorder, copd, lung chronic obstructive disease, lung disease, chronic obstructive, obstructive chronic lung disease, obstructive chronic pulmonary disease, obstructive lung disease, chronic, pulmonary disease, chronic obstructive, pulmonary disorder, chronic obstructive Medline specific subject headings: MSK – exp Musculoskeletal Diseases/ (this also covers: musculoskeletal disease, musculoskeletal diseases, orthopedic disorder, orthopedic disorders, bone disease, bone diseases, disease bone, diseases bone, cartilage disease, cartilage diseases, chondromalacia, chondromalacias, fasciitides, fasciitis, fascitides, fasciitis, deformities foot, deformities metatarsal, deformity, foot, deformity metatarsal, foot deformities, foot deformity, metatarsal deformities, metatarsal deformity, foot disease, foot diseases, deformities hand, deformity hand, hand deformities, hand deformity, disease, jaw,diseases, jaw disease, jaw diseases, arthropathies, arthropathy, joint disease, joint diseases, muscle disorder, muscle disorders, muscular disease, muscular diseases, myopathic condition, myopathic conditions, myopathies, myopathy, abnormalities musculoskeletal, abnormality musculoskeletal, musculoskeletal abnormalities, musculoskeletal abnormality, disease rheumatic, diseases rheumatic, rheumatic disease, rheumatic diseases, rheumatism) Diabetes – Diabetes, Gestational/ (this also covers: diabetes gestational, diabetes mellitus gestational, diabetes pregnancy induced, diabetes pregnancy-induced, gestational diabetes, gestational diabetes mellitus, pregnancy-induced diabetes) – Diabetes Mellitus, Type 1/ (this also covers: autoimmune diabetes, brittle diabetes mellitus, diabetes autoimmune, diabetes, juvenile-onset, diabetes mellitus brittle, diabetes mellitus insulin dependent, diabetes mellitus insulin-dependent, diabetes mellitus insulin-dependent 1, diabetes mellitus juvenile onset, diabetes mellitus juvenile-onset, diabetes mellitus ketosis prone, diabetes mellitus ketosis-prone, diabetes mellitus sudden onset, diabetes mellitus sudden-onset, diabetes mellitus type 1, diabetes mellitus type I, diabetes type 1, iddm, insulin dependent diabetes mellitus 1, insulin-dependent diabetes mellitus, insulin-dependent diabetes mellitus 1, juvenile onset diabetes, juvenile-onset diabetes, juvenile-onset diabetes mellitus, ketosis-prone diabetes mellitus, sudden-onset diabetes mellitus, type 1 diabetes, type 1 diabetes mellitus) – Diabetes Mellitus, Type 2/ (this also covers: adult-onset diabetes mellitus, diabetes, maturity-onset, diabetes mellitus, adult onset, diabetes mellitus, adult-onset diabetes mellitus ketosis resistant, diabetes mellitus ketosis-resistant, diabetes mellitus maturity onset, diabetes mellitus maturity-onset, diabetes mellitus non insulin dependent, diabetes mellitus non-insulin-dependent, diabetes mellitus noninsulin dependent, diabetes mellitus noninsulin-dependent, diabetes mellitus slow onset, diabetes mellitus slow-onset, diabetes mellitus stable, diabetes mellitus type 2, diabetes mellitus type ii, diabetes type 2, ketosis-resistant diabetes mellitus, mody, maturity onset diabetes, maturity onset diabetes mellitus, maturity-onset diabetes, maturity-onset diabetes mellitus, niddm, non-insulin-dependent diabetes mellitus, noninsulin dependent diabetes mellitus, noninsulin-dependent diabetes mellitus, slow-onset diabetes mellitus, stable diabetes mellitus, type 2 diabetes, type 2 diabetes mellitus COPD – exp Pulmonary Disease, Chronic Obstructive/ (this also covers: airflow obstruction chronic, airflow obstructions, chronic, coad, copd, chronic airflow obstruction, chronic airflow obstructions, chronic obstructive airway disease, chronic obstructive lung disease, chronic obstructive pulmonary disease, chronic obstructive pulmonary diseases, pulmonary disease chronic obstructive, asthma copd overlap syndrome, asthma chronic obstructive pulmonary disease overlap syndrome, asthma-copd overlap syndrome, asthma-copd overlap syndromes, asthma-chronic obstructive pulmonary disease overlap syndrome, overlap syndrome, asthma-copd, bronchitis, chronic, chronic bronchitis, centriacinar emphysema, centriacinar emphysemas, centrilobular emphysema, centrilobular emphysemas, emphysema centriacinar, emphysema, centrilobular, emphysema focal, emphysema panacinar, emphysema panlobular, emphysema pulmonary, emphysemas centriacinar, emphysemas centrilobular, emphysemas focal, emphysemas panacinar, emphysemas panlobular, emphysemas pulmonary, focal emphysema, focal emphysemas, panacinar emphysema, panacinar emphysemas, panlobular emphysema, panlobular emphysemas, pulmonary emphysema, pulmonary emphysema) Cockrane specific subject headings: Diabetes – (“Diabetes Mellitus” OR “Type 1 Diabetes Mellitus” OR “Type 2 Diabetes Mellitus” OR “Disorder Associated With Type 2 Diabetes Mellitus”) MSK – ( “Musculoskeletal Disorder” OR “Low back pain” OR “Shoulder Pain” OR “Neck Pain” OR “Musculoskeletal Symptom” OR “Musculoskeletal and connective tissue conditions NEC” OR “Hip Pain” OR “Knee Pain” OR “Fracture ” OR “Osteoporosis” OR “Osteoarthritis” “Foot Pain”) COPD (“Chronic Obstructive Pulmonary Disease” OR “Chronic Bronchitis” OR “Pulmonary Emphysema” ) Free Text: MSK – Musculoskeletal condition* or MSK or musculoskeletal disease* or orthop?edic disorder* or musculoskeletal complaint or musculoskeletal disorder or musculoskeletal symptom* or nonspecific mechanical lower back pain* or cervical neck pain* or osteoarthritis or fractures or hip condition* OR joint condition* or knee condition* or osteoporosis or elbow pain* or shoulder pain* or wrist pain* ankle pain* foot pain* COPD – chronic obstructive pulmonary disease or copd or emphysema or chronic bronchitis Diabetes – Diabetes or diabetes adj5 type 1 or diabetes adj5 type 2 or LADA or MODY |
PICOS Element | Key words | Search terms |
---|---|---|
Interventions | Personalised Care Shared Decision Making Supported Self-Management Social Prescribing Personalised Care and Support Planning Choice. | General subject heading: *social support/ or *community support/ or *self care/ or *decision Making, Shared/ or *patient education as Topic/ Embase specific subject headings: – *peer group/ or *peer support/ (this also covers: peer nomination, peer nominations, peer relation, peer relations, peer relationship, peer relationships, peer support* – self care/ (this also covers: self management, self treatment, self−management, self−nurturance, selfcare, selfmanagement, selftreatment) – patient education as Topic/ (this also convers: education patient, patient education as topic, patient medication knowledge) Medline specific subject headings: – *peer group/ or *peer influence/ (this also covers: influence peer, peer influence, peer pressure, pressure peer) -*social support/ (this also covers: care social, online social support, online social supports, perceived social support, perceived social supports, social care, social support, social support online, social support perceived, social supports online, social supports perceived, support online social, support perceived social, support social, supports perceived social) – *community support/ (this also covers: community support, community supports, support community) Cochrane specific subject headings: – (“Social Support OR Shared Decision Making OR Self Care OR Self-care tools OR Self-help therapy OR Self-care Practice OR Patient Education OR Self-care Patient Education OR “Peer support” OR “Self-management” OR “Personalized Care Planing” OR “Personalized medicine” OR “Prescribing” OR “Provision Of Patient Decision Aid” OR “Decision coaching” OR “Community-Based” OR “Self supported care” OR “Self-help material” OR “promotion of self care” OR “self-care interventions” or Computer-based self-management” Free text: – Personalis* Care OR Shar* Decision Making OR Support* Self-Management OR Social Prescribing OR Support Planning OR Patient choice OR tailor* support or tailor* care or bespoke treatment OR Bespoke care OR Bespoke support OR support planning OR Collective decision making OR joint decision making OR mutual decision making OR Personal decision making OR Assisted self-management OR SSM or supported self-management or supported self-care OR assisted self-care OR self-management support OR self-management education OR peer support OR social prescribing OR health coaching OR Community based support OR community support OR person centred care OR informal support OR right to choose OR Personal health budget* OR PHB OR integrated personal budget* OR IPB OR enabl* choice OR Personal health budget* OR patient activation or decision support tool*or patient decision aids or support tools or brief decision aids or DSTs or health education or peer network or anticipatory care or clinical assessment service* or referral management centre* or single point of access or Link Worker* or community-based approaches or PCSP or community activation |
PICOS Element | Key words | Search terms |
---|---|---|
Outcomes of interest | Health utilisation (admissions, A&E, GP attendance etc) and impact on clinical outcomes, wellbeing outcomes, patient experience & safety. | Outcomes: health utilisation, clinical outcomes, wellbeing outcomes, patient experience & safety General subject headings: – *hospitalization/ (this also covers: hospital stay, short stay hospitalization) – *patient admission/ (this also covers: admission hospital, admitting department hospital, hospital admittance, hospital admitting department, hospital admitting service, hospital admitting unit, patient admission, waiting list, waiting lists, admission patient, admission voluntary, admissions patient, admissions voluntary, patient admission, patient admissions, voluntary admission, voluntary admissions – *patient readmission/ (this also covers:, readmission, readmission rate, readmissions, 30 day readmission, 30 day readmissions, hospital readmission, hospital readmission unplanned, hospital readmissions, hospital readmissions unplanned, patient readmission, readmission hospital, readmission patient, readmission thirty day, readmission unplanned, readmissions hospital, thirty day readmission, thirty day readmissions, unplanned hospital readmission, unplanned hospital readmissions, unplanned readmission, unplanned readmissions) – *Quality of Life/ (this also covers; health related quality of life, HRQL, life quality – Wellbeing/ or emotional well-being/ or physical well-being/ or psychological well-being/ or health care utilization/ Embase specific subject headings: – *patient participation/ (this also covers: patient involvement, patient participation rate) or *patient satisfaction/ or *patient preference/ *patient Safety/ Medline specific subject headings: – *patient acceptance of health care/ (this also covers: acceptability of health care, acceptability of healthcare, acceptors of health care, care acceptor health, care acceptors health, care nonacceptor health, care nonacceptors health, health care acceptability, health care acceptor, health care acceptors, health care nonacceptor, health care nonacceptors, health care seeking behavior, health care utilization, healthcare acceptabilities, healthcare acceptability, healthcare patient acceptance, healthcare patient acceptances nonacceptors of health care, patient acceptance of health care, patient acceptance of healthcare, utilization health care – *patient compliance/ (this also covers: adherence client, adherence patient, client adherence, client compliance, client compliances, compliance client, compliance patient, compliance therapeutic, compliance treatment, cooperation patient, non adherent patient, non-adherence patient, non-adherent patient, non-adherent patients, non-compliance patient, nonadherence patient, noncompliance patient, patient adherence, patient compliance, patient cooperation, patient non adherence, patient non compliance, patient non-adherence, patient non-adherent, patient non-compliance, patient nonadherence, patient noncompliance, therapeutic compliance, therapeutic compliances, treatment compliance, treatment compliances – *patient dropouts/ (this also covers: dropout patient, dropouts patient, patient dropout, patient dropouts) – *patient participation/ (this also covers: activation patient, empowerment patient, engagement patient, involvement patient, participation patient, participation rate patient, participation rates patient, patient activation, patient empowerment, patient engagement, patient involvement, patient participation, patient participation rate, patient participation rates – *patient satisfaction/ or *treatment refusal/ (this also covers: anesthesia refusal, anesthesia refusals, elopement patient, elopements patient, patient elopement, patient elopements, patient refusal of treatment, refusal anesthesia, refusal treatment, refusal of treatment, refusals anesthesia, refusals treatment, treatment refusal, treatment refusals) Cochrane specific subject headings: General: “Hospitalization” OR “Quality of Life” OR “Patient Satisfaction Score” OR “Patient care” OR “Health Care Utilization ” OR “Quality Of Wellbeing Scale” OR “General well-being ” OR “Patient Satisfaction” COPD: “Weight Loss” OR “Weight Gain” OR “Exercise” OR “Pulmonary Rehabilitation” OR “Difficulty Breathing” OR “Psychological wellbeing” Diabetes “HbA1c” OR “HbA1c level” OR “hypoglycaemia” OR “Hyperglycaemia” OR “diabetic foot” or “Diabetic neuropathy” OR “weight gain” OR weight loss or exercise level* OR “diabetic complications” MSK “Pain” OR “Depression” OR “Anxiety” OR “Visual Analog Score” Free text: General Health utilisation or hospital admission* or A&E admission* or GP attendance or clinical outcome* or wellbeing outcome* or patient experience* or patient safety or patient satisfaction or hospital utilisation or patient wellbeing or personal wellbeing or remission or patient response or quality of life or empowering patient* or unplanned service or patient confidence or patient knowledge or community resilience or psychological wellbeing or anxiety or depression or PAM score* or self efficacy or Patient Reported Outcome Measures or PROMs or European Quality of Life or 5D 3L or ICEpop CAPability measure for Older people or ICECAP O or Adult Social Care Outcome* or ASCOF or Patient Reported Experience Measure* or PREMS or Overall Patient Experience Score* or OPES or patient discharge or excess bed days or DToCs or delayed transfer of care or discharge to assess or d2a pathway or improved symptoms Diabetes HbA1c or hypoglycaemia or HYPERGLCAEMIA or urine acr or diabetic foot disease or Macrovascular complications or Microvascular complications or Diabetic neuropathy or peripheral neuropathy or Nephropathy, weight gain* or weight loss or exercise level* MSK pain level* or subacute pain or chronic pain or acute pain or depression or anxiety or psychological wellbeing or quality of life or VAS score or MSK HQ COPD weight loss or exercise tolerance or exercise intolerance or health-related quality of life or health resource or pulmonary rehabilitation or breathlessness or flare-up* or persistent cough or persistent coughing |
PICOS Element | Key words | Search terms |
---|---|---|
Setting/Study design | Preference for a UK setting and publication date within the last 10 years. |
Appendix 3: Definitions of outcomes
Diabetes
We have defined clinical outcomes for personalised care interventions on diabetes to include:
- Glycaemic control, as measured by changes in HbA1c levels
- Blood pressure
- Lipid profile
- Body weight
- Complications of diabetes, such as neuropathy, nephropathy, retinopathy, and cardiovascular disease.
We have defined wellbeing outcomes for personalised care interventions on diabetes to include:
- Quality of life: This includes measures of physical, emotional, and social wellbeing.
- Mental health: This includes measures of anxiety, depression, and stress.
- Self-efficacy: This includes the patient’s belief in their ability to manage their diabetes and the extent to which they feel in control of their condition.
- Health-related behaviours: This includes measures of healthy eating, physical activity, and other lifestyle changes that can help manage diabetes.
we have defined healthcare utilisations for personalised care interventions on diabetes to include:
- Hospitalization rates: The number of hospitalizations due to diabetes-related complications, such as cardiovascular disease, kidney disease, or foot ulcers.
- Emergency department visits: The number of emergency department visits due to diabetes-related complications, such as hypoglycemia or hyperglycemia.
- Outpatient visits: The number of outpatient visits for diabetes-related care, such as check-ups, diabetes education, or medication management.
- Medication adherence: The proportion of patients who take their diabetes medication as prescribed by their healthcare provider.
- Cost-savings: Refers to reducing expenses and improving efficiency without sacrificing the quality of care.
We have defined patient experience outcomes to include:
- Empowerment: This refers to a patient’s sense of control over their diabetes management and can be assessed through surveys or questionnaires.
- Patient knowledge:
- Perceived support: This refers to a patient’s perception of the support they receive from healthcare providers, family members, and peers, and can be assessed through surveys or questionnaires.
- Patient satisfaction: This includes measures of patient satisfaction with the care they receive and their overall experience of the intervention.
We have defined patient safety for personalised care interventions on diabetes to include:
- Hypoglycaemia: This refers to abnormally low blood sugar levels and can be a serious and potentially life-threatening complication of diabetes management. The frequency and severity of hypoglycaemic events may be monitored.
- Diabetic ketoacidosis (DKA): This is a serious complication that can occur when there is a shortage of insulin in the body. The frequency and severity of DKA episodes may be monitored.
- Adverse drug reactions: Diabetes management often involves the use of medications such as insulin and oral hypoglycaemic agents, which can have side effects. Adverse drug reactions may be monitored to ensure patient safety.
- Foot ulcers: Diabetes can cause nerve damage and poor circulation, which can increase the risk of foot ulcers. Monitoring the frequency and severity of foot ulcers can help to prevent complications.
MSK:
For our research, we have defined clinical outcomes for personalised care interventions on MSK conditions to include:
- Reduced pain: One of the primary goals of personalized care interventions for MSK conditions is to alleviate pain. Clinical outcomes may include reduced pain intensity, decreased frequency of pain episodes, and improved pain-related quality of life.
- Improved function: Personalized care interventions may also aim to improve physical function and mobility. Clinical outcomes may include improved range of motion, increased strength and endurance, and enhanced ability to perform daily activities.
- Reduced medication use: Depending on the personalized care intervention, some individuals may experience a decrease in their reliance on pain medication, which can have important clinical outcomes such as reduced risk of adverse effects and improved overall health.
Wellbeing Outcomes:
For our research, we have defined wellbeing outcomes for personalized care interventions on MSK conditions to include:
- Improved quality of life: Personalized care interventions can help individuals with MSK conditions to improve their quality of life by reducing pain, improving physical function, and increasing participation in activities that are meaningful to them.
- Increased self-efficacy: Personalized care interventions can help individuals with MSK conditions to feel more in control of their condition and more confident in their ability to manage their symptoms.
- Enhanced sense of empowerment: Personalized care interventions can help individuals with MSK conditions to feel more empowered to make decisions about their care and take an active role in managing their condition.
- Improved social functioning: MSK conditions can impact an individual’s social life, leading to isolation and reduced social functioning. Personalized care interventions can help individuals to improve their social functioning by reducing pain, improving physical function, and increasing participation in social activities.
Healthcare utilisation:
For our research, we have defined healthcare utilisation outcomes for personalized care interventions on COPD conditions to include:
- Reduced use of healthcare services: Personalized care interventions can help individuals with COPD to better manage their symptoms, potentially reducing the need for frequent healthcare visits, such as emergency room visits, hospitalizations, or specialist consultations.
- Improved adherence to treatment: Personalized care interventions can help individuals with COPD to adhere to their treatment plan, which can lead to better outcomes and reduce the need for additional healthcare services.
- Reduced healthcare costs: Personalized care interventions can help to reduce the overall cost of healthcare for individuals with COPD by improving outcomes and reducing the need for costly healthcare services.
- Improved healthcare efficiency: Personalized care interventions can help to improve the efficiency of healthcare delivery for individuals with COPD by reducing the need for unnecessary or ineffective treatments and improving the use of healthcare resources.
Patient Safety:
For our research, we have defined patient safety outcomes for personalized care interventions on COPD conditions to include:
- Reduced risk of adverse events: Personalized care interventions can help to reduce the risk of adverse events, such as medication errors, falls, or other complications, by providing individualized care that is tailored to the specific needs of each patient.
- Improved communication: Personalized care interventions can help to improve communication between patients and healthcare professionals, reducing the risk of miscommunication and misunderstandings that can lead to adverse events.
- Increased patient involvement: Personalized care interventions can help to increase patient involvement in their own care, which can help to reduce the risk of adverse events and improve patient safety.
- Enhanced monitoring and follow-up: Personalized care interventions can include careful monitoring and follow-up to ensure that patients are responding well to treatment and to identify any potential safety concerns.
Appendix 4: Summary tables for high-impact interventions
The tables below lists the most impactful personalised care outcomes across the research studied. If the numerical data was unavailable within a systematic review, we searched the primary paper they cited for this information. In such cases, the primary authors have been referenced in the ‘Outcome’ column. More caution should be given to these outcomes/impacts, as their results may not have been validated as part of a wider meta-analysis.
Paper ID | Intervention | Study Type | Primary outcome | Numerical impact |
---|---|---|---|---|
209 | Telehealth (nurse-led) | MA | Reduction in number of hospital admissions | -4.1% (telehealth group had 152 out of 640 (23.8%), usual face-to-face group of participants there was 218 out of 780 (27.9%). |
217 | Patient empowerment | SR | Reduction in hospital admissions (1 study relating to Pharmacist-led medication therapy, Erku, 2017) | -52.1% in number of admissions |
84 | Telehealth | MA | + adherence = + cost-effectiveness | 1% adherence = $5.42 |
+ adherence = + cost-effectiveness | 1Hg decrease = $7.39 | |||
11 | Patient education | SR | One study reported increase in medical adherence compared to usual care (Negarandeh, 2011) | + 20% adherence (adherence to dietary: 3.63 vs 5.87 and 6.15 out of maximum 9 score) and medication regimens (4.32 vs 6.73 and 7.03 out of maximum 8) |
Two studies reported significant increase in knowledge scores in diabetes following the intervention (Swavely 2013, Negarandeh, 2011) | Diabetes knowledge test: 84% score in IG vs 40.7 % in CG. (Swavely 2013) Mean end point knowledge score (29.41 in control vs 35.32 in intervention (Negarandeh, 2011) | |||
17 | Peer support | MA | Reduction of Hba1c | Pooled MD of -0.57% [95% CI: −0.78 to −0.36] |
30 | Patient education (Culturally Tailored Diabetes Educational Intervention) | MA | Improved Glycaemic control | Pooled ES of glycaemic control in RCTs with CTDEI was -0.29 (95% confidence interval, -0.46 to -0.13) |
57 | Telehealth | SR | A meta-analysis of mobile phone interventions found they increased glycaemic control (Liang, 2013) | Reduced HbA1c values by a mean of 0.5% over a median of 6 months follow-up duration [6mmol/mol; 95% confidence interval, 0.3–0.7% (4–8 mmol/mol)] |
66 | Telehealth (web-based interventions) | MA | Five studies with outcome data for depression showed reduced feelings of depression (58, 53, 60, 59, 50) | The pooled mean difference between the IG and CG’s depression score was -0.31 (95% confidence interval) |
Six studies that reported on distress found it was reduced | The pooled mean difference between intervention and control on distress scores was -0.11 (-0.38 to 0.16 | |||
84 | Telehealth | MA | MA reported a significant reduction in blood pressure compared to UC. | -3.74 mmHg (sbp), -2.37 mmHg (dbp) |
352 | Patient education | MA | Thirty-one studies assessed the impact of the interventions on fasting blood glucose (27,29-33,37-44,46-60,62,63) | 71% of studies observed that the educational programs produced statistically significant improvements in FBG |
352 | Patient education | MA | Thirty-one studies assessed the impact of the interventions on hba1c (27,29-33,37-44,46-60,62,63) | 59% of studies observed that the educational programs produced statistically significant improvements in hba1c |
352 | Patient education | MA | Thirty-one studies assessed the impact of the interventions on fasting blood glucose (27,29-33,37-44,46-60,62,63) | 57% of studies observed that the educational programs produced statistically significant improvements in fasting blood sugar |
391 | Patient empowerment | MA | Compared to routine care, empowerment-based intervention is associated with reduced glycated haemoglobin levels | SMD -0.20; (95% CI -0.31 to -0.08; Z=3.40, P<.001, I2=42%) |
391 | Patient empowerment | MA | Compared to routine care, empowerment-based intervention was associated with increased diabetes empowerment scores | SMD 0.24; (95% CI 0.10–0.37; Z=3.42, P<.001, I2=0%) |
391 | Patient empowerment | MA | Compared to routine care, empowerment-based intervention was associated with increased diabetes knowledge scores | SMD 0.96; (95% CI 0.55–1.36; Z=4.61, P<.001, I2=80%) |
448 | Telehealth (computer based) | SR | In a meta-analysis of 11 trials, computer-based interventions have shown benefits for glycaemic control | Pooled effect on HbA1c: -2.3 mmol/mol or -0.2% (95% confidence interval (CI) -0.4 to -0.1; P = 0.009; 2637 participants; 11 trials). |
Improved disease knowledge (Lo, 1996) | 10.9 to 14.3 on diabetes knowledge scale | |||
Increases patient empowerment (Lorig, 2010) | +0.021(PAM Score) | |||
Improved patient knowledge (Quinn, 2008) | Knowledge of food choices compared with the control group (91% versus 50%) | |||
Improves self-efficacy (Quinn, 2008) | Diabetes self-care questionnaire (100% versus 75%). | |||
Telehealth (mobile phone) | SR | Meta-analysis of three mobile phone-based interventions found a statistically and clinically significant reduction in HbA1c (Liang, 2011) | MD in HbA1c -5.5 mmol/mol or -0.5% (95% CI -0.7 to -0.3); P < 0.00001; 280 participants; three trials). | |
189 | Telehealth (mobile phone) | MA | RCTs compared Smartphone Technologies with usual diabetes care among T2DM patients and reported a significant reduction in HbA1c | Pooled weighted mean difference: -0.51%; 95% confidence interval: -0.71% to -0.30%; p < 0.001), favouring ST intervention. The pooled weighted mean difference was -0.83% in patients with T2DM <8.5 years and -0.22% in patients with T2DM ≥8.5 years, with significant subgroup difference (p = 0.007). |
84 | Telehealth (interactive digital interventions -IDIs) | MA | Overall, IDIs significantly reduced SBP | WMD -3.74mmHg [95% confidence interval (CI) -2.19 to -2.58] with no heterogeneity observed (I-squared¼0.0%, P¼0.990). |
84 | Telehealth (interactive digital interventions -IDIs) | MA | For DBP, four out of six studies indicated a greater reduction for intervention compared to controls, with no difference found for two. | WMD of -2.37mmHg (95% CI -0.40 to -4.35) was found, but considerable heterogeneity was noted (I-squared¼80.1%, P¼<0.001). |
Increased self-efficacy | Correlated with Positive Outcome Expectations (r = 0.30, P = 0.037) and Diabetes Self-Management and Diabetes Quality of Life for Youths (r = 0.43, P = 0.002) | |||
209 | Telehealth (nurse-led) | MA | The intervention groups of community-dwelling older adults significantly improved in overall QoL. | SMD 0.12; (95% CI 0.03 to 0.20; P=0.006; I2=21%) |
The intervention groups of community-dwelling older adults significantly improved in overall self-efficacy | SMD 0.19; (95% CI 0.08 to 0.30; P<.001; I2=0% ) | |||
The intervention groups of community-dwelling older adults significantly improved in overall depression levels. | SMD –0.22; (95% CI –0.36 to –0.08; P=.003; I2=89% ) | |||
214 | Telehealth (wearable insole) | SR | Reduced diabetic foot ulcer occurrence (Abbot, 2019) | 86% reduction at 18month follow-up |
Telehealth (digital medicine offering) | SR | DMO resulted in a statistically greater SBP reduction than usual care (Frais, 2017) | Mean –21.8, SE 1.5 mm Hg vs mean –12.7, SE 2.8 mmHg; mean difference –9.1, 95% CI –14.0 to –3.3 mm Hg) and maintained a greater reduction at week 12 | |
217 | Patient Empowerment | SR | Seven studies (24,26,35,38,39,41,42)with meta-analysable data on blood pressure showed statistically significant differences between control in favour of interventions. | The pooled results for SBP MD was −5.13 [95% CI: −9.42, −0.84] (P = .02) |
Seven studies (24,26,35,38,39,41,42)with meta-analysable data on blood pressure showed statistically significant differences between control in favour of interventions. | The pooled results for DBP indicated that there is a statistically significant difference in the outcomes of mean difference (MD) −4.28 [95% CI: −7.18, −1.37] (P = .004) | |||
Four studies were included in a meta-analysis (24,26,41,42). The pooled results indicate that there is a small, statistically significant difference in the outcomes between intervention and control groups in terms of hba1c. | Overall effect size of −0.59 (95% CI: −0.72, −0.47] (P < .00001) | |||
227 | Telehealth (nurse-led) | MA | Pooled intervention effects from 2 studies showed a significant improvement in the systolic blood pressure of patients through Telerehabilitation. | MD 10.48; (95% CI, MD 1.52; 95% CI) |
The pooled SMD indicates significant positive effect on enhancing the self-care behavior of patients with diabetes when compared with conventional face-to-face nursing consultations | SMD 1.20; (95% CI 0.55-0.84; P<.001; heterogeneity: X2 4=46.3; I2=91%; P<.0) | |||
352 | Patient education | MA | Meta-analysis of 20 randomized controlled trials (3,094 patients) indicated that the programs produced a reduction in hba1c | SMD −0.31% (95% CI −0.48% to −0.14%). |
391 | Patient empowerment | The meta-analysis showed that compared to routine care, empowerment based intervention was associated with reduced glycated hemoglobin levels | SMD -0.20 (95% CI -0.31 to -0.08; Z=3.40, P<.001, I2=42%) | |
Five studies [8,12,13,16,18] measured the psychosocial self-efficacy by the scores of Diabetes Empowerment Scales (DES). Patient empowerment improved significantly in the intervention group as compared with the control | SMD 0.24; (95% CI 0.10–0.37; Z= 3.42, P<.001) | |||
Four studies[7,12,14,18] provided the scores of diabetes knowledge after Intervention.The score of diabetes knowledge was significantly higher in the intervention group than the control | SMD 0.96 (95% CI 0.55–1.36; Z=4.61, P<.0) |
Paper ID | Intervention | Study Type | Outcome | Numerical impact |
54 | Decision support | MA | Participants receiving care with the DAs had greater gains in general knowledge compared with UC, with no evidence of a treatment interaction with any of the sociodemographic characteristics analysed. | 62% vs 45%; P<0.0001 |
Patients who used the DAs were found to know their personalized risk (knowledge of risk) more often than those receiving UC | 50% vs 20%; P<0.0001 | |||
Decisional conflict was lower for patients in the DA arm as compared with UC across all sociodemographic groups. There were no significant treatment interactions between sociodemographics. | 13 (intervention) vs 18 (control) points | |||
Clinicians encouraged patient empowerment significantly more often when using decision support. | 39 (Intervention) vs 21 (Control) | |||
158 | Telehealth | SR | Reduction in school absenteeism (Armbrust et al., 2017) | 43% to 14% |
Telehealth (iCanCope) | Reduction in pain intensity (Lalloo et al., 2020) | 1.73-point reduction on 1-10 scale | ||
160 | Telehealth (FitBit) | SR | Walking time (Amorim et al., 2019) | Increase of 183.1 min/week |
164 | Self-management education (booster sessions) | MA | significant reduction in pain catastrophizing in patients with CMP after a self-management intervention | SMD 20.42 (95% CI) |
212 | Telehealth | MA | Reduction in pain | 5.7% reduction |
Digital-based structured SMPs vs health education condition | Favours digital SMPs (SMD 0.26; 95% CI) | |||
Reduction in pain | 5.7% reduction | |||
Increase in physical function | 5.07% improvement | |||
Improved quality of life | 0.17 SDs higher | |||
349 | Self-management education | SR | Reduction in pain | SMD between groups was ‐0.26 (95% CI ‐0.44 to ‐0.09); mean reduction of 0.8 points on VAS Scale |
Intervention | Study Type | Outcome | Numerical impact |
---|---|---|---|
Health coaching | MA | Significant beneficial impact on quality of life | SMD = −0.69, 95% CI: −1.28, −0.09, p =.02, from k = 4 |
significant reduction in COPD-related hospital admissions | (OR = 0.46, 95% CI: 0.31, 0.69, p =.0001, from k = 5) | ||
Blended self-management | MA | Reduction in exacerbation frequency | Relative Risk =0.38; 95% CI 0.26-0.56 |
Significant reduction in BMI | d=0.81; 95% CI 0.25-1.34 | ||
Large effect was found on QoL | SMD=0.81; 95% CI | ||
Nutritional support | MA | Significantly greater increases in mean total protein and energy intakes | (1.94 ± 0.26 kg, P < 0.001 |
Self-management interventions | MA | HRQoL Assessed with: St. George’s Respiratory Questionnaire adjusted total score. Scale from: 0 to 100 Note: lower scores indicate better HRQoL | 2.86 points lower (4.87 lower vs 0.85 lower) |
Lower risk of emergency department visits | -0.52 (95% CI) | ||
Reduced SGRQ score, indicating better quality of life | -2.86 (95% CI) | ||
Improvement in exercise capability | MD of 45.14 meters in walking (95% CI 9.16 to 81.13; Analysis 2.13). | ||
Self-management interventions (action plans) | Statistically significantly lower risk for at least one respiratory-related hospital admission | OR 0.69, 95% CI 0.51 to 0.94 | |
Self-management education | SR | Activity levels signficantly improved: Six studies, with 772 participants, measured exercise capacity using the six-minute walking test (6MWT) and could be included in the meta-analysis | Pooled MD of 45.14 meters reached the MCID of 25 meters and therefore is considered clinically relevant (Holland 2010). |
self-management interventions including exacerbation action plans with a smoking cessation programme | contributed to significant improvements in HRQoL (Lenferink 2017). | MD from usual care of ‐2.69 points (95% CI ‐4.49 to ‐0.90; 1,582 participants; 10 studies; high‐quality evidence). |